From data to insights: unlocking the value of analytics | FEBRUARY 2021 Enterprises are drowning in data, yet many of them struggle to extract insights from that data to deliver value. As the artificial intelligence (AI) and machine-learning revolution intensifies, long-term investors must ensure the companies they back are seizing the opportunities locked away in those ever-proliferating troves of data.
Judy Wade
Managing Director and Head of CPP Investments’ San Francisco office.
Kelly Shen
is Senior Managing Director and Chief Technology & Data Officer at CPP Investments.
Frederic Laluyaux
a long-time tech entrepreneur, is CEO of Aera Technology.
Katie Doyle
chairs the innovation and sustainability committee on the board of grocery retailer Ahold Delhaize and is former CEO of Swanson Health Products.
Igor Taber
is SVP of Corporate Development and Strategy at DataRobot.
Ali Ghodsi
is co-founder and CEO of Databricks, a data, AI, and analytics platform.
Melinda White
is CEO of 5G wireless infrastructure provider, Transit Wireless, a company in CPP Investments’ portfolio.
Judy Wade recently led a panel discussion with six executives developing or adopting complex data and analytics solutions. The conversation made clear that, while the right technology and approach matters, the most important variable is a culture that embraces digital analytics. Here are select insights from the discussion.
Capturing value from data
Conversations about generating insights through advanced analytics usually revolve around four characteristics of data, the so-called four Vs: volume (the amount of data generated), velocity (the speed of incoming data), variety (differing types and sources of data) and veracity (data quality and accuracy).
The last V is especially important. Without veracity, companies are unable to take advantage of ‘X Analytics’ – the ability to run sophisticated analytics on a broad swath of data of any type, in any format, no matter where it resides. As enterprises combine more and more disparate datasets, instead of creating data lakes that can be tapped for insights, they often produce complex data swamps from which insights seldom emerge.
The problem is worse now than it was 20 years ago, when most of an organization’s data resided in a single vendor’s database, says Ghodsi.
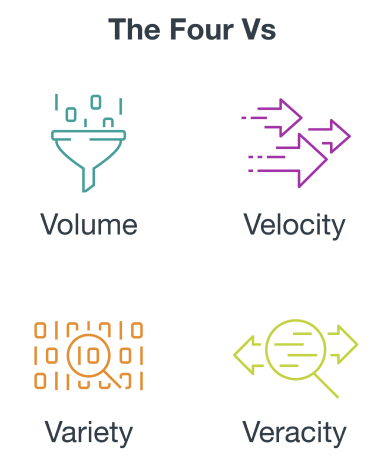
Today, that information is dispersed among numerous applications and attempts to collate it create major quality issues.
As artificial intelligence and machine learning approach mainstream adoption, veracity is being redefined by validation and explainability, or interpretability. AI systems have historically been perceived as black boxes. Business leaders often had limited understanding of the inputs and how the outputs were derived. For users to understand, trust and manage AI, validating the underlying data and providing transparency into the model is critical.
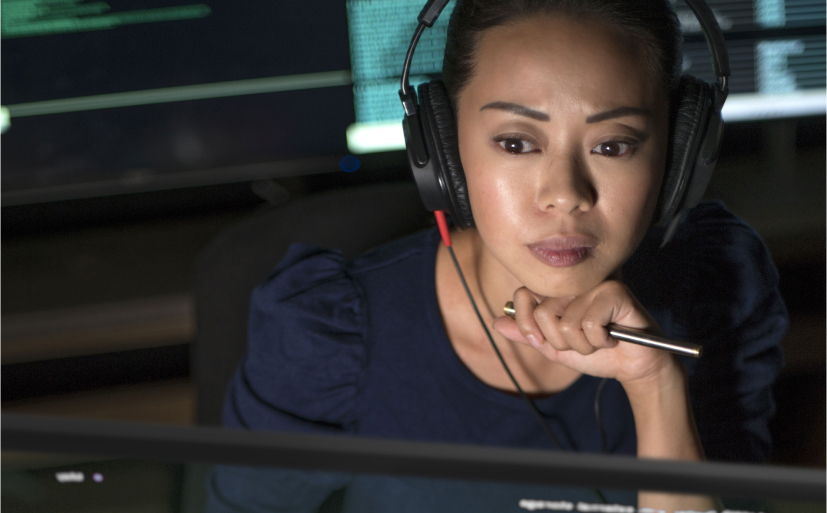
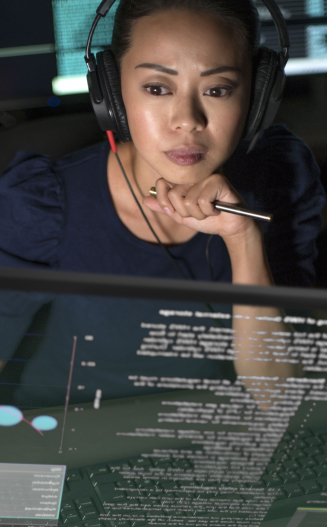
“The secret sauce to doing good AI is the data itself,” says Ghodsi, “and every little dataset that you add might improve your accuracy”— but only if it is made comparable. Subtle inconsistencies, such as different date formats, can ruin an analysis and the ability to get actionable business recommendations. Being very clear about the goal is key to cutting through the complexity.
“For us, it’s understanding very clearly the outcomes, what the customer aims to achieve,” says White, whose firm provides communication networks and smart data products for some of North America’s largest transit systems, including the New York City subway. “One thing we learned early in the process is, it’s not just about what we develop as product, it’s about being able to integrate with existing data.” Successful automation of insights also requires consistent definition of metrics. “If you want to deploy artificial intelligence on top, you need to understand what the data means and how to transform it into a set of actionable insights,” says Laluyaux. He points out, for example, that different approaches to tracking on time and in full (OTIF) statistics — a common logistics measurement — across data sources can undermine the validity of machine-learning output.
What Laluyaux describes is not uncommon. Wade recalls that when two major grocers merged operations and combined data, the analytics seemed wrong. It took the team a long time to figure out that one dataset tracked inventory daily and the other weekly.
Future-proofing your data decisions
Leveraging open-source software can help organizations avoid getting locked into proprietary solutions that over time may become cumbersome legacy technologies. “Once a [solution provider] has your data, they lock you in,” says Ghodsi. “It’s a moat for them.” By relying on open-source solutions and the cloud, companies can future-proof their data capabilities by preserving the ability to migrate to new AI platforms. Building a strong data analytics foundation from the start is essential, the panelists agree.
"Shen recommends creating a common goal that everyone in the organization can rally around."
"Data science should not be a bolt-on later"
– Ghodsi
Digital-native companies such as Uber, Facebook and Airbnb were deliberate about ensuring their data was set up for machine-learning and actionable recommendations, which gives them a big advantage. “They built their businesses around that asset,” says Laluyaux. “If you make the right choices now, you might buy yourself more time and flexibility in the next five or ten years.”
Companies that fail in this regard are apt to waste time and money trying to build a data-analytics capability on the side. What many people don’t realize about AI, Taber says, is that “most of the models built by data scientists never make it into production, in part because these specialists are forced to cobble together disparate tools. In order to truly unlock the potential of AI, you need a single system of record to build, deploy and manage your AI.”
Data democracy versus centralization
Natural tension exists between the need to centralize governance of data privacy and compatibility, and simultaneously make the analytics tools available to those running individual businesses or functions. Taber’s company, for example, focuses on democratizing data science and AI by expanding the pool of people who can create, manage, and consume insights. However, too much democracy can turn into anarchy. When Doyle ran a global wellness business, “the culture was that everyone could tinker,” she recalled.
“You could play with different datasets, put out promotions and see what happened.” But the lessons from those experiments were not captured centrally. She addressed that by establishing central control over data capture and governance policies and then decentralizing the access to analytics tools to enable innovative uses.
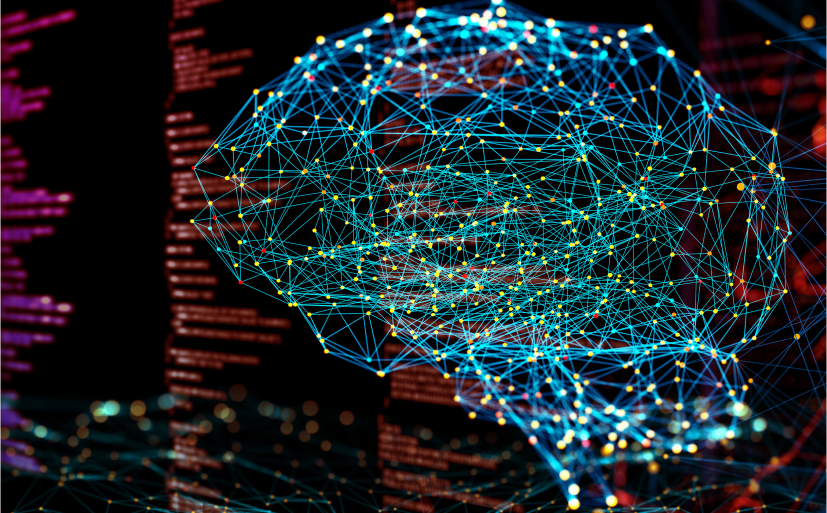
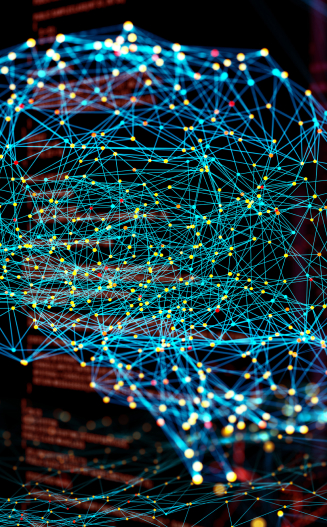
No impact without cultural transformation
Ultimately, the biggest barriers to extracting value from AI and machine-learning investments are not technical but cultural.
Mindsets and processes need to be fundamentally transformed, but many large enterprises have gone through several prior waves of organizational re-engineering — outsourcing, process optimization, migration to the cloud — and now suffer what Laluyaux calls “transformation fatigue.” “They struggle to put this new digital capability at the core of what they do and to reorganize their people and their processes around that capability,” he says. That makes it critical for leaders to stay on message, consistently making the case for transformation, even as they are working through the nitty gritty of digitizing operations. “It’s hard,” says Shen. “You have all the things that you have to do every day, and then you’re also trying to help people think differently, imagine working differently, introducing automation.”
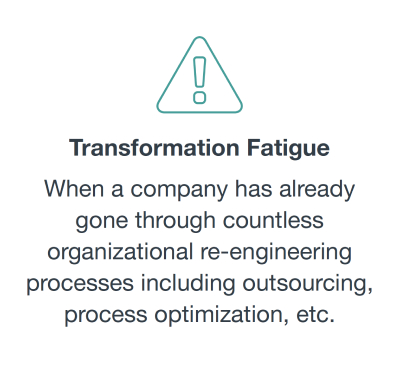
"Shen recommends creating a common goal that everyone in the organization can rally around."
"Data science should not be a bolt-on later"
– Ghodsi
“That way, we’ve put it out there in black and white,” she says. “We’ve said that we’re going to do this, we’ve committed to it, we’ve made it known to everyone who works with us.” In a legacy organization, that full embrace of analytics requires an enormous change to existing belief systems. “A lot of people will still want to rely on their own intuition or their own experience, rather than the insights and line of sight that you get from analytics,” says White. Overcoming that simple hurdle requires a huge cultural change.
Driving adoption on the frontlines
AI and machine learning will see limited uptake without buy-in from P&L leaders. These key operators hold much of the organizational power and often feel that their resources are better spent on initiatives with stronger track records.
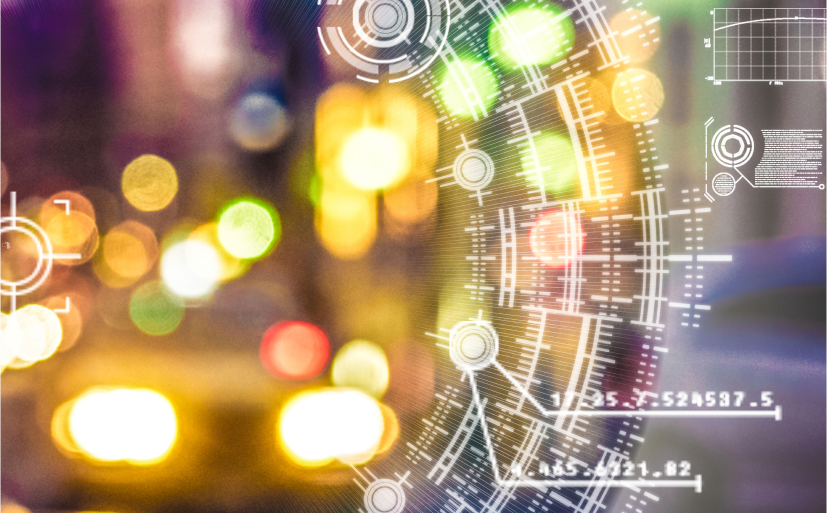
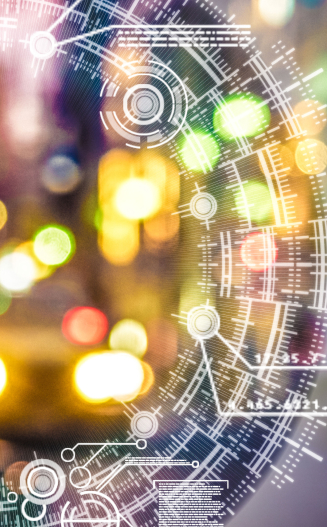
“You need to help them believe in [analytics],” says Doyle. Building a set of case examples, along with a road map for executing them, can help allay the sense of overwhelming and frightening change, she adds. Getting a few early victories on the board can also help quell dissent. For that reason, Taber recommends focusing initial AI projects on applications with easily measured impact. Laluyaux concurs, adding, “it’s rational to go to the highest-value, lowest-complexity uses, but the important thing is to start with use cases where you can clearly articulate the value generated.”
Fostering organization-wide adoption also requires some one-on-one engagement. “Spend time talking to those whose decisions are going to be impacted on a daily basis by the application you’re implementing,” Laluyaux advises, because convincing them is more important than convincing the board or the CEO; although leadership buy-in is critical to establishing an agenda that everyone in the enterprise can rally around. At Ahold Delhaize, two of the top candidates for CEO succession have been put in charge of the company’s data-analytics project, says Doyle. “They have to be part of the recommendations of how we will build and use it and the levels of investment we make. And they have to present that to the board.”
‘Pace of change will never be as slow as it is today’
It’s still early days in the AI revolution, but a subset of industries and companies are rapidly maturing. One silver lining in the catastrophic COVID-19 pandemic is that many previous skeptics have been convinced of the value of digital analytics — not only in predicting the pandemic’s impact but in highlighting shifts in consumer behaviour. As a result, adoption of AI and machine learning is rising. “The pace of change will never be as slow as it is today,” says Doyle.
“In retail, the haves and the have-nots are very clear right now,” she adds, pointing out that some major winners, which had invested in analytics and e-commerce capabilities, now stand out sharply against a backdrop of widespread bankruptcies. “When it’s clear six months from now who survives and who doesn’t, everyone is going to find religion and accelerate the pace exponentially.” Digital natives have an edge in data and analytics, but incumbents bring their own competitive advantages: massive stores of data collected over decades, customer relationships, and industry know-how. While being a first mover has both risks and benefits, Taber argues that in AI, the advantages are more pronounced than in some other technological transitions.
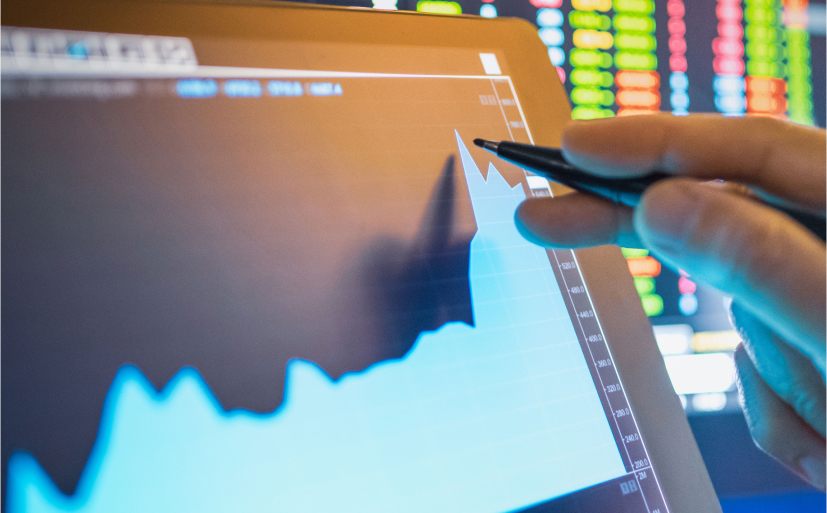
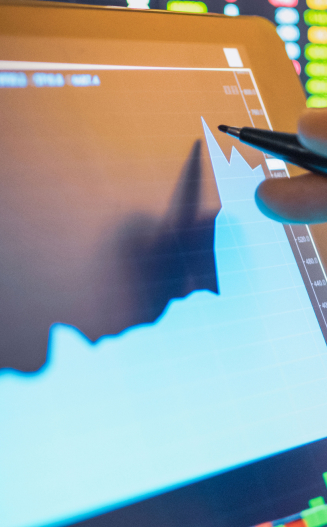
“The better you are at working with your data, the more accurate your models are,” says Taber, “and that gap between the haves and have-nots is going to increase.” Laluyaux believes the acceleration that will leave latecomers far behind is already underway. “We’re in the middle of it,” he says. “We’re in the eye of the storm, so we’re not feeling the wind.”
He cites a Harvard professor’s observation that executives are moving from FOMO (fear of missing out) to COMO (certainty of missing out). “They have a certainty they will miss out if they don’t do something,” he says. “They just don’t know what that something is. [But] if they don’t do it, they won’t survive, and they know it.”
Sustaining Strength: How Strategic Capital Supports Canadian Resource Leadership
Canada is an energy powerhouse—and Canadian Natural Resources Limited is one of its strongest engines.
Seeding the Future: Radical Ventures and the Rise of Canadian AI
CPP Investments manages approximately $114 billion in assets across Canada. Its holdings in hundreds of companies span sectors such as
Four Minutes with Heather Tobin
Heather Tobin, Senior Managing Director & Global Head of Capital Markets and Factor Investing, shares insights on the role of disciplined